If you consume online content, use social media, or just generally not live under a rock, you’ve probably come across AI-generated images often in your waking life. Some are realistic enough to trick you into thinking they’re a human creation or a photograph. Others, like the infamous AI hand salad, have probably given you a good laugh — and peace of mind knowing that AI is lightyears away from taking over the world.
But regardless of your stance on AI-generated graphics, it’s hard to deny that the technology is a fascinating, albeit controversial, leap for humanity. Machines’ ability to convert text into relevant and realistic graphic outputs is still nothing short of awe-inspiring, especially when you consider auto-generated works that closely mimic human artistic style and even convey emotion.
In this article, we’ll do our best to uncloak the mysterious workings of AI image models. We’ll outline the technologies used in the text-to-image process, introduce a few popular AI image tools, and address the controversies surrounding machine-generated art.
{toc}
The technology and process behind AI image generation
In simple terms, generative AI relies on the data it's been trained on to produce images. The AI’s neural networks learn to discern image patterns during training, then use this pattern-recognition skill to put together images in response to text prompts.
This process makes use of sophisticated systems, called “architectures” in the industry. The two main architectures designed to generate AI images are General Adversarial Networks (GAN) and diffusion models, and they function somewhat differently. Meanwhile, Natural Language Processing models (NLPs) help the AI process and understand human text inputs.
Let’s take a deeper look at these technologies below.
GANs make use of competing networks to produce realistic images

GAN is an earlier architecture used by AI image models, one that’s lately been challenged by diffusion models. However, GANs are still used in several generative AI products, including GANPaint Studio, Artbreeder, Deepart, and RunwayML.
The GAN architecture is made up of two neural networks. One, the Generator, produces images based on its training datasets. The other, the Discriminator, is taught to distinguish between real images and the Generator’s output. When the Generator creates an image, the Discriminator looks it over and either flags it as authentic or as a fake. If the Discriminator labels the image a fake, the Generator undergoes an upgrade to improve its generation quality.
On the other hand, if the Discriminator falls for it and thinks the Generator’s image is real, it becomes due for an upgrade itself. This adversarial process continues in perpetuity, causing Generator to produce increasingly realistic images.
Diffusion models refine random noise into coherent images
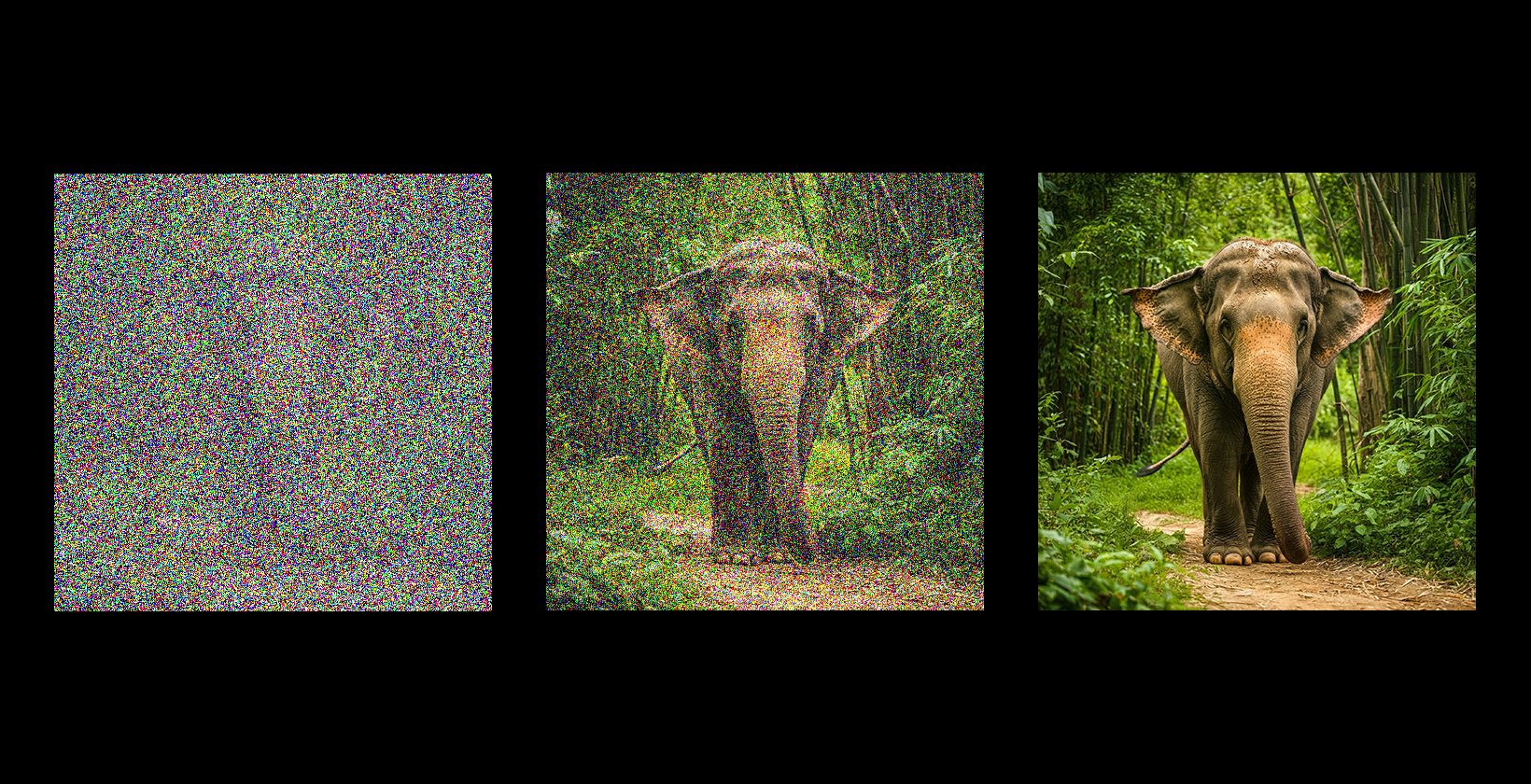
Diffusion models are a newer architecture used by popular image generators, like Dall-e and Midjourney. Diffusion models also rely on their training datasets to create images in response to prompts.
The process goes like this.
Presented with a real image, the model learns to add noise to it by distorting it or adding random pixels (hence the term “diffusion”). This first stage is called “forward diffusion,” and the point of it is to teach the model how to reconstruct the image from a degraded state.
Next, the model analyzes how the forward diffusion process changed the image into an incomprehensible mess. Once this analysis is complete, the reverse diffusion process starts, and the model learns to remove the noise to restore the image to its original likeness — much like putting together a puzzle.
After the model is trained on these forward and reverse diffusion exercises, it becomes ready to generate images based on human inputs. When the user describes the image they want to create, the model starts with the diffused state and gradually gets rid of the noise until an image reflecting the text input remains.
NLP and LLMs help AI understand user text inputs
Whether a generative AI product employs GANs or diffusion models, it needs to have a way to understand human inputs before it can get to work “drawing” the output. And that’s where Natural Language Processing (NLP) and Large Language Models (LLMs) step in to lend a hand. Simply put, NLP is a system crucial to the workings of LLMs because it allows the latter to understand text prompts. In turn, an LLM guides the image generator to a graphic output that illustrates the user’s text input.
For instance, if you enter the prompt “Elephant wearing a hat,” the NLP system will break the phrase down into separate chunks — “elephant,” “wearing,” and “hat.” Then, it will convert the bits of text text into numeric sequences. These sequences enable the LLM to understand the individual words and their contextual meaning. Armed with this information, the AI’s image generation architecture gets to work producing an output that matches your description.
Popular AI image creation apps
With the evolution of AI image-generation capabilities and a growing demand for this technology, a number of image creation apps have entered the market. Some of the most popular and reputable AI image apps are:
- Dall-e
- Midjourney
- Stable Diffusion
- Adobe Firefly
Dall-e
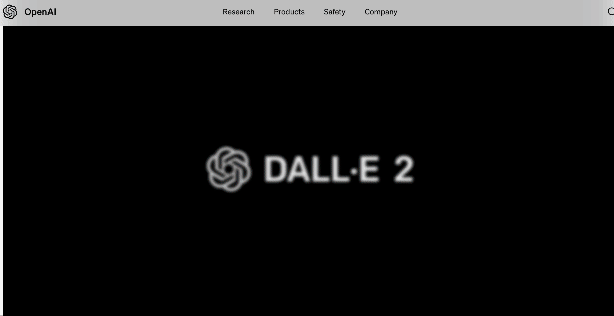
Developed by ChatGPT’s parent company, OpenAI, Dall-e is one of the most sophisticated image creation apps out there. Its newest iteration is powered by a diffusion model, and is capable of combining unrelated concepts and text into realistic, high-quality images. You can access Dall-e as a standalone app in OpenAI’s suite of products, or through a ChatGPT account.
Midjourney
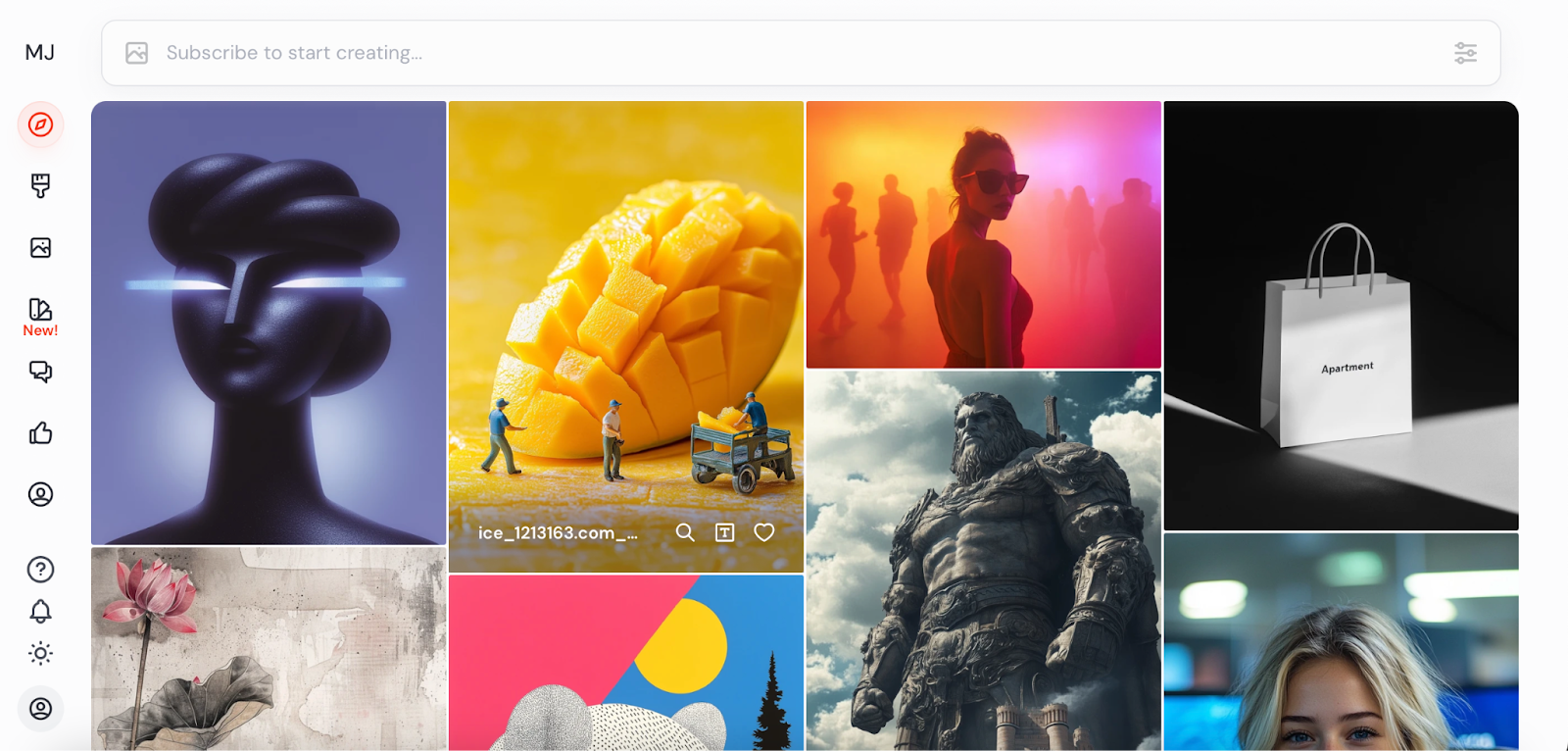
Midjourney is an acclaimed AI image model that stands out for its heavy focus on artistic creativity and its ability to replicate various art styles. The program utilizes a proprietary neural network architecture, which experts believe is based on a diffusion model. You can access Midjourney through a Discord or Google account, and subscription options range between $8 and $96 per month.
Stable Diffusion
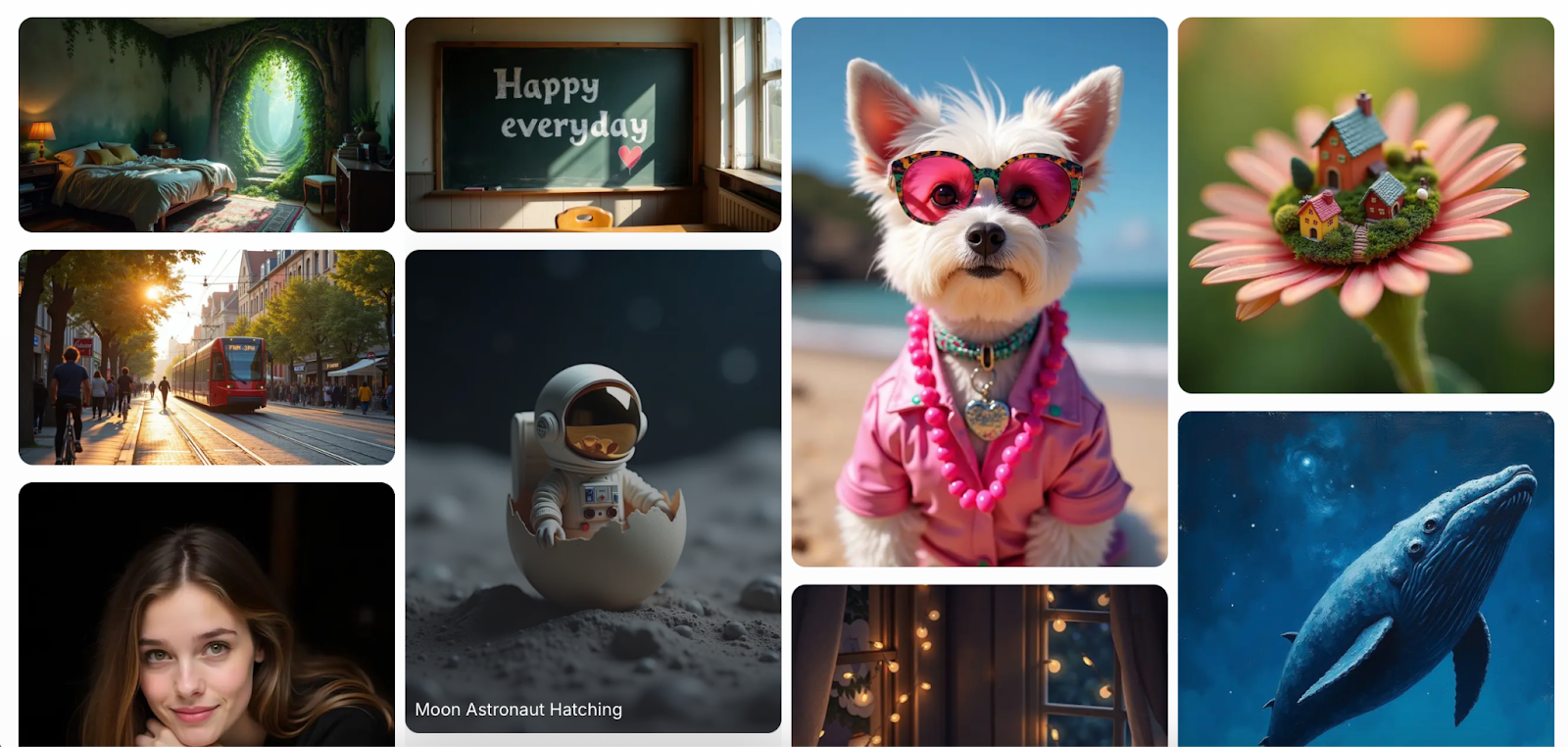
Stable Diffusion is an open-source image creation program that can run on your hardware and offers extensive customization options. At its core is a latent diffusion model that’s capable of converting text to pictures and editing existing images. Its customizability makes Stable Diffusion a hit among graphic artists with strong programming skills and a solid grasp on machine learning.
Adobe Firefly
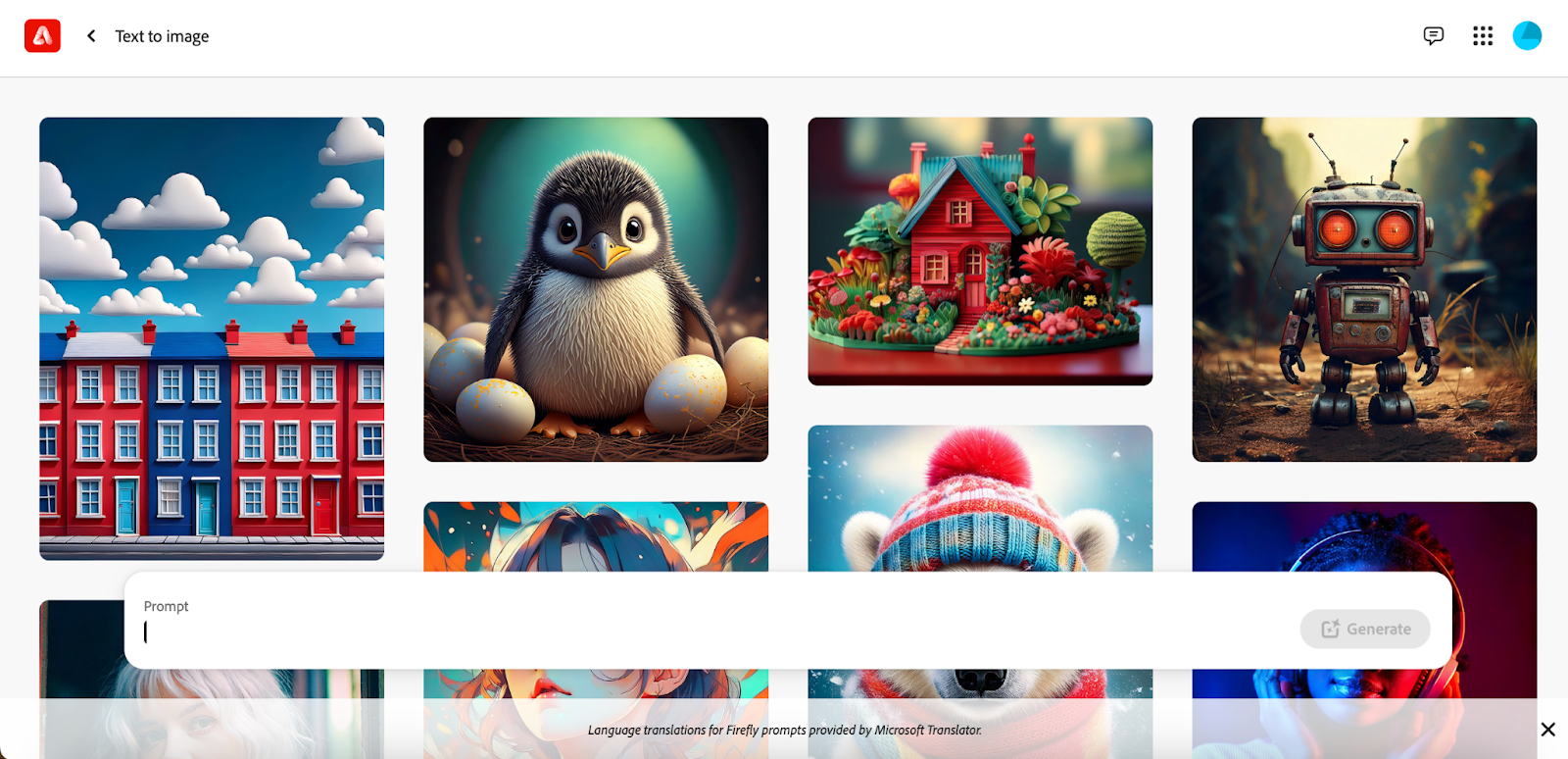
Adobe Firefly is an AI image generator that integrates into Adobe’s suite of creative tools, including Illustrator, Photoshop, and others, and allows you to create new images and alter existing ones. This integration with Adobe’s tools makes Firefly an appealing choice for creative professionals who use the Adobe ecosystem. To generate graphic content, Firefly uses a diffusion model.
Common applications of AI image generation
AI image generation is now used in many fields where images and artwork were once exclusively in the human domain. Among other applications, AI-produced illustrations can be found in:
- Content marketing and advertising
- Art
- Entertainment
- Presentations
- Design and prototyping
- Medical imaging
Content marketing and advertising
AI-generated images have been a godsend for content marketers and advertisers. Creating graphics used to be a tedious, expensive task that involved engaging graphic artists and putting each image through multiple revision cycles. Thanks to the power of AI, the process became faster and more efficient.
Whether the resulting images are of better quality than their human-made counterparts is up for debate (and outputs can range from captivating to cringeworthy). That said, here are a few examples of successful AI-based marketing campaigns from famous brands:
Coca-Cola’s Create Real Magic campaign
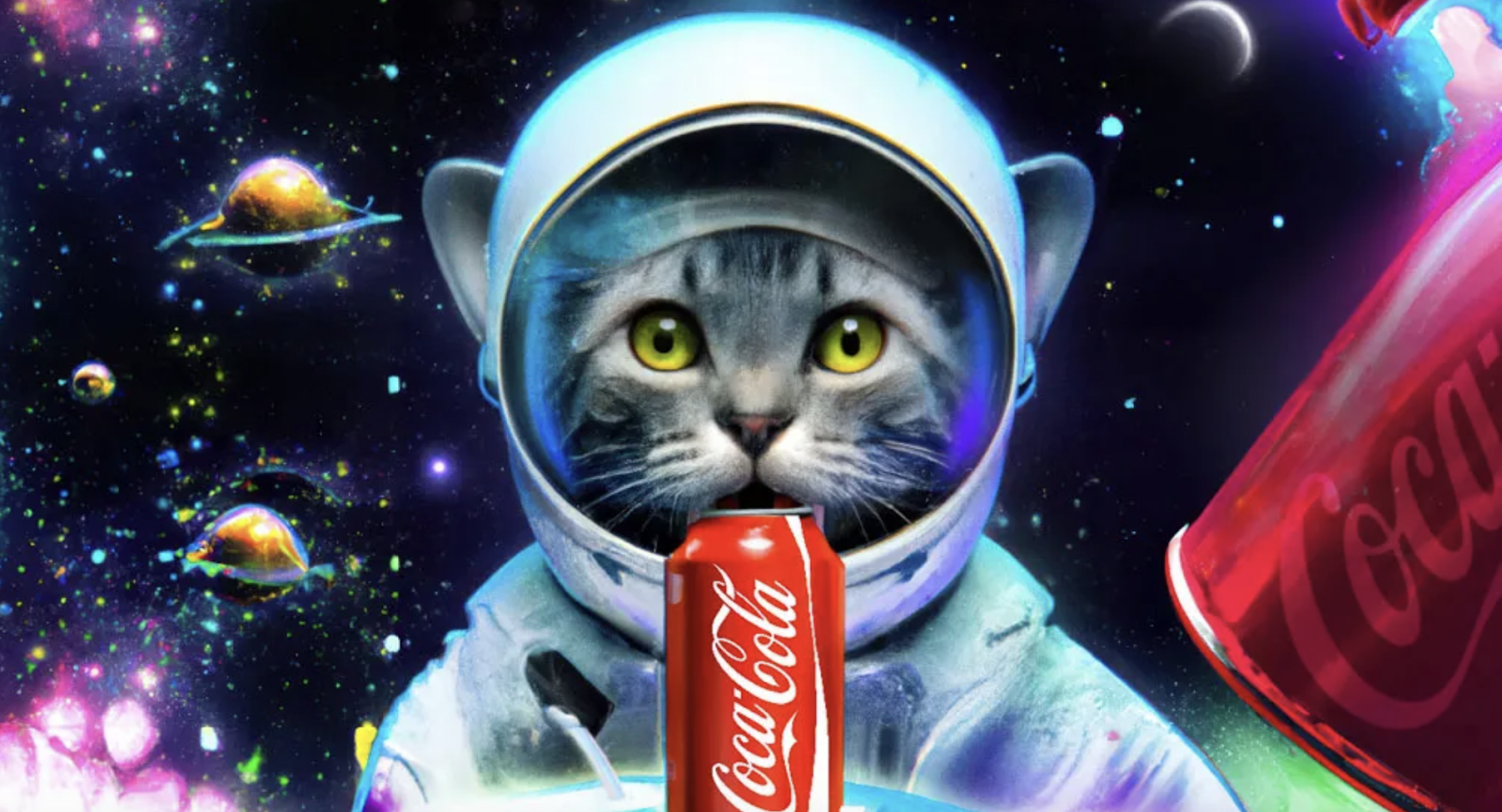
Coca-Cola’s Create Real Magic platform allowed users to generate AI image content using Dall-e and ChatGPT, as well as the company’s branding and graphic assets. Coca-Cola saw an opportunity in using AI and user-generated content together to push the boundaries of creativity in marketing. A number of artists participated in the campaign, and 30 of the participants were selected to collaborate with Coca-Cola in producing marketing material.
Nutella Unica labels
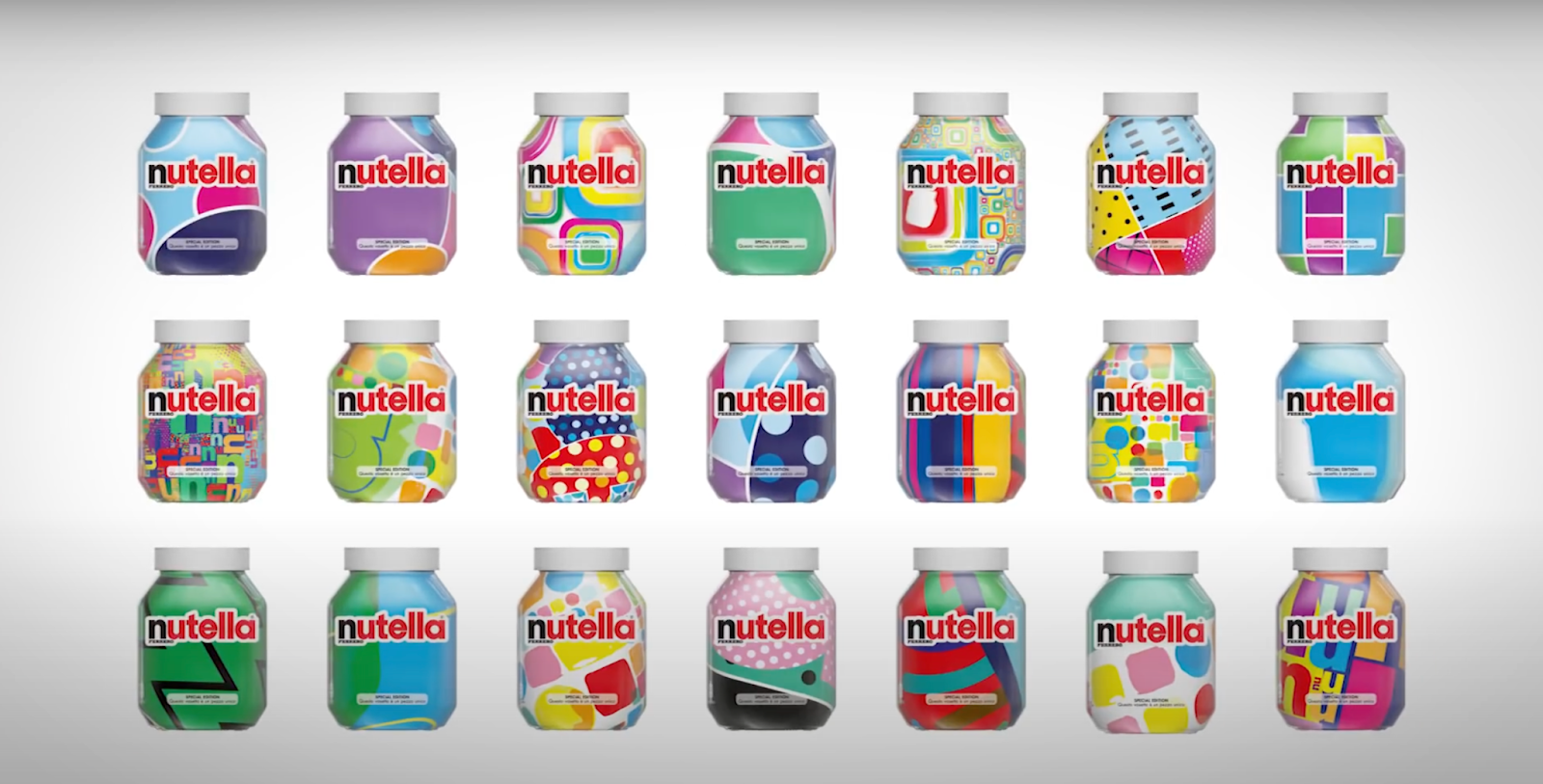
Like Coca-Cola, Nutella and the ad agency it hired (Ogilvy & Mather Italia) turned to AI to get a major creativity boost for its marketing campaign in Italy. According to Nutella’, the intent of the campaign was to “individualize” its product by making the design of each jar label unique. Now, we’re talking about 7 million jars — each with one-of-a-kind AI-produced graphic design on its label. What would’ve been an impossible undertaking with human effort alone became reality thanks to an AI model that generated each design.
BMW: projecting AI art onto cars

BMW ventured in the world of auto-generated graphics when it used generative AI to produce, in its own words, “The Ultimate AI Masterpiece.” The piece was produced by an AI model pretrained on 50,000 of the world’s most revered paintings and contemporary art. BMW then projected the output onto its 8 Series Gran Coupé. The point of this marketing campaign was to draw parallels between 900 years’ worth of humanity’s artistic achievements, advancements in artificial intelligence, and BMW’s knack for creativity and innovation.
Art
The first artificial intelligence program to dip its robot toes into the world of art was AARON. AARON was a software conceived by British painter Harold Cohen way back in 1973. Cohen was able to connect AARON to drawing mechanisms that could interpret its commands and produce images on paper. Here’s a photo of AARON at work, with Cohen in the background (courtesy of Kate Vass Gallery):
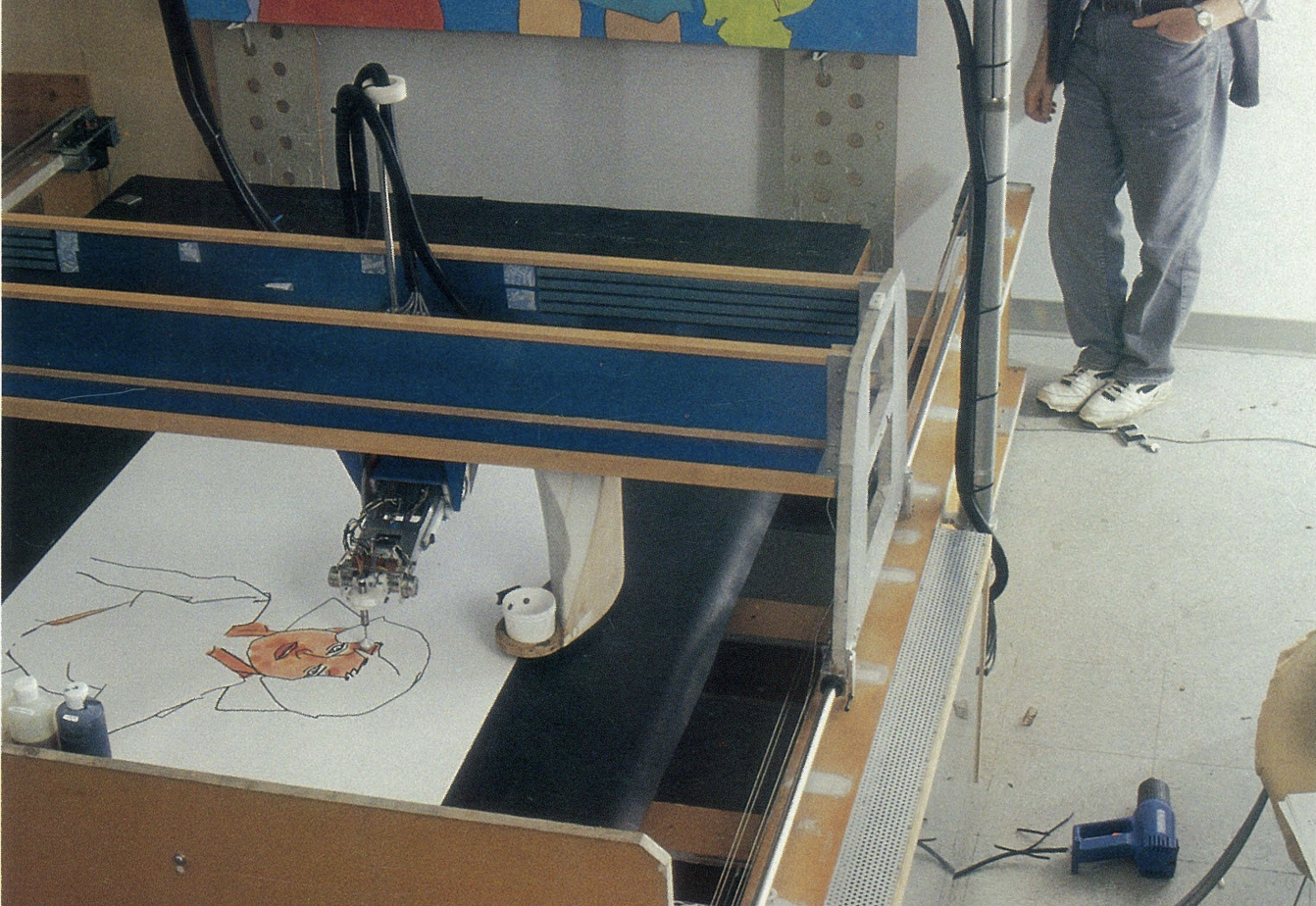
To generate the images, AARON relied on algorithms that its creator had programmed. At the time of its inception in the 1970s, AARON could produce nothing but monochromatic drawings that had to be colored by Cohen. By the 1990s, the machine was capable of choosing colors and generating images that resembled human beings and nature.
Decades after AARON made its debut, AI image generators evolved significantly, and GANs were the mainstream architecture used to auto-generate art. In 2018, a painting produced by the Obvious art collective using GAN architecture fetched a juicy $432,500 at Christie’s. The painting’s title, Edmond de Belamy, pays homage to the inventor of GAN networks, Ian Goodfellow — “belle ami” means “good friend” in French, a reference to the scientist’s last name.
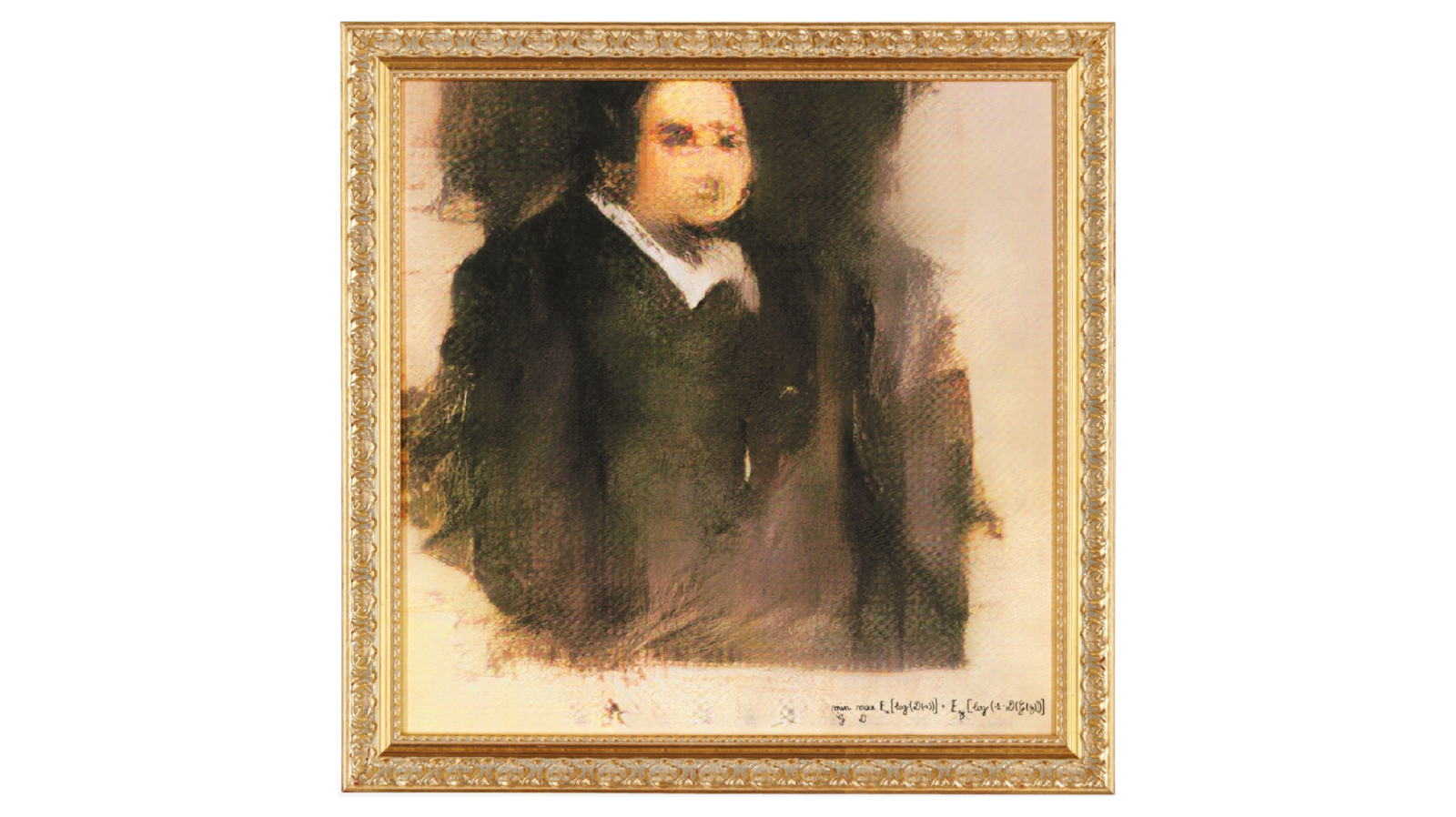
Only a few years later in 2022, a work produced by Midjourney stunned the world by clinching the winning spot at the Colorado State Fair annual art competition. Produced by diffusion model architecture, Théâtre D'opéra Spatial stirred controversy by winning a title previously reserved for human artists.

Following these important milestones, AI-based art has continued to evolve and proliferate. As it becomes more mainstream, AI art brings forth more and more pressing questions surrounding authenticity, intellectual property, and the role of humans in the realm of creativity. (We touch on these thorny subjects below.)
Entertainment
Apart from making a splash in the art scene, AI-generated images have also found their way into film. In the last several years, a few animated films have been produced entirely with Dalle-made AI images, like The Frost:
Likewise, AI graphics are often used in traditional cinematography. For example, the recently released film Late Night With the Devil made use of generative AI to produce interstitial ads that appear in the movie.
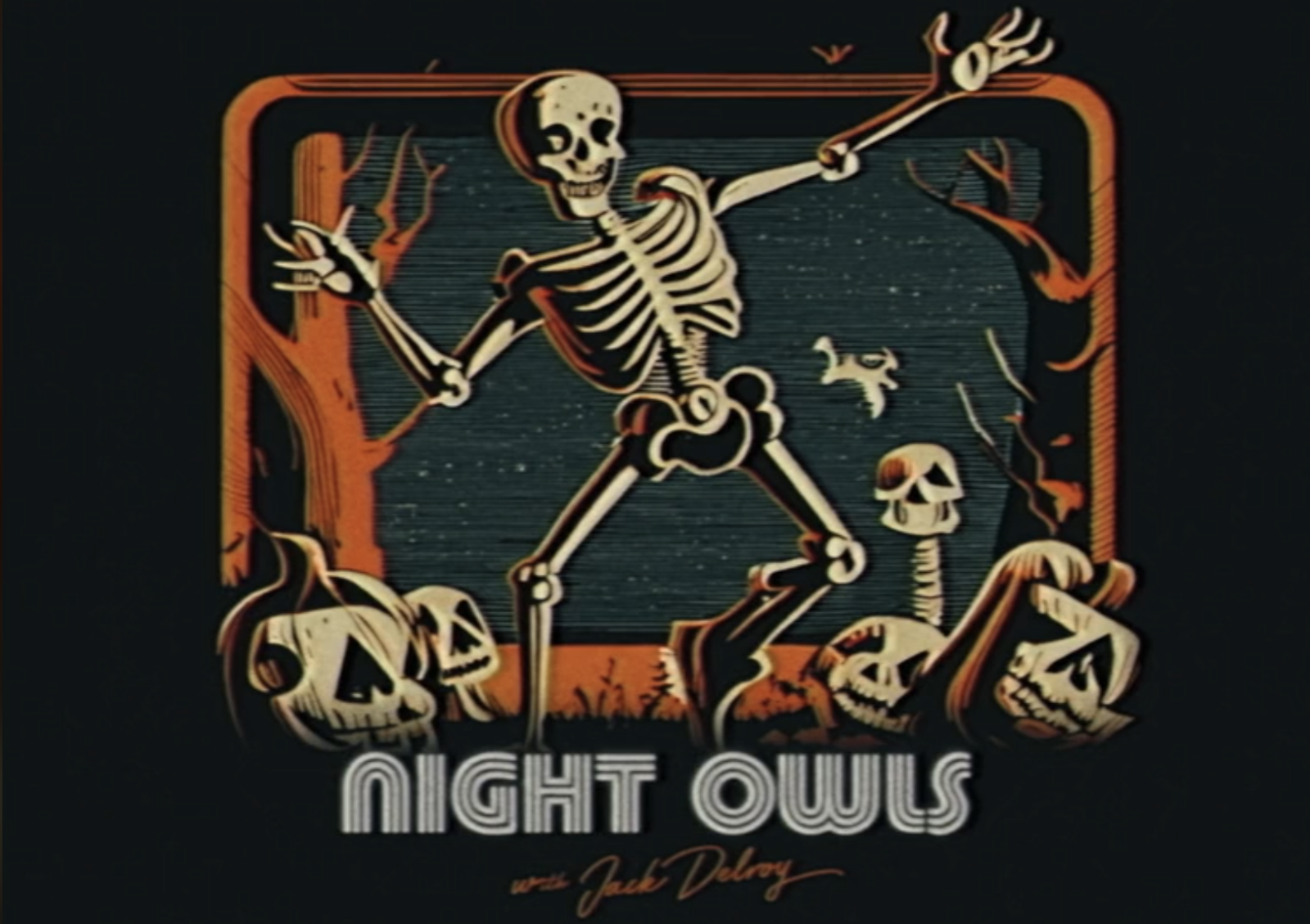
Despite the relatively minor role of AI in this production, audiences were profoundly agitated by its use.
AI presentations
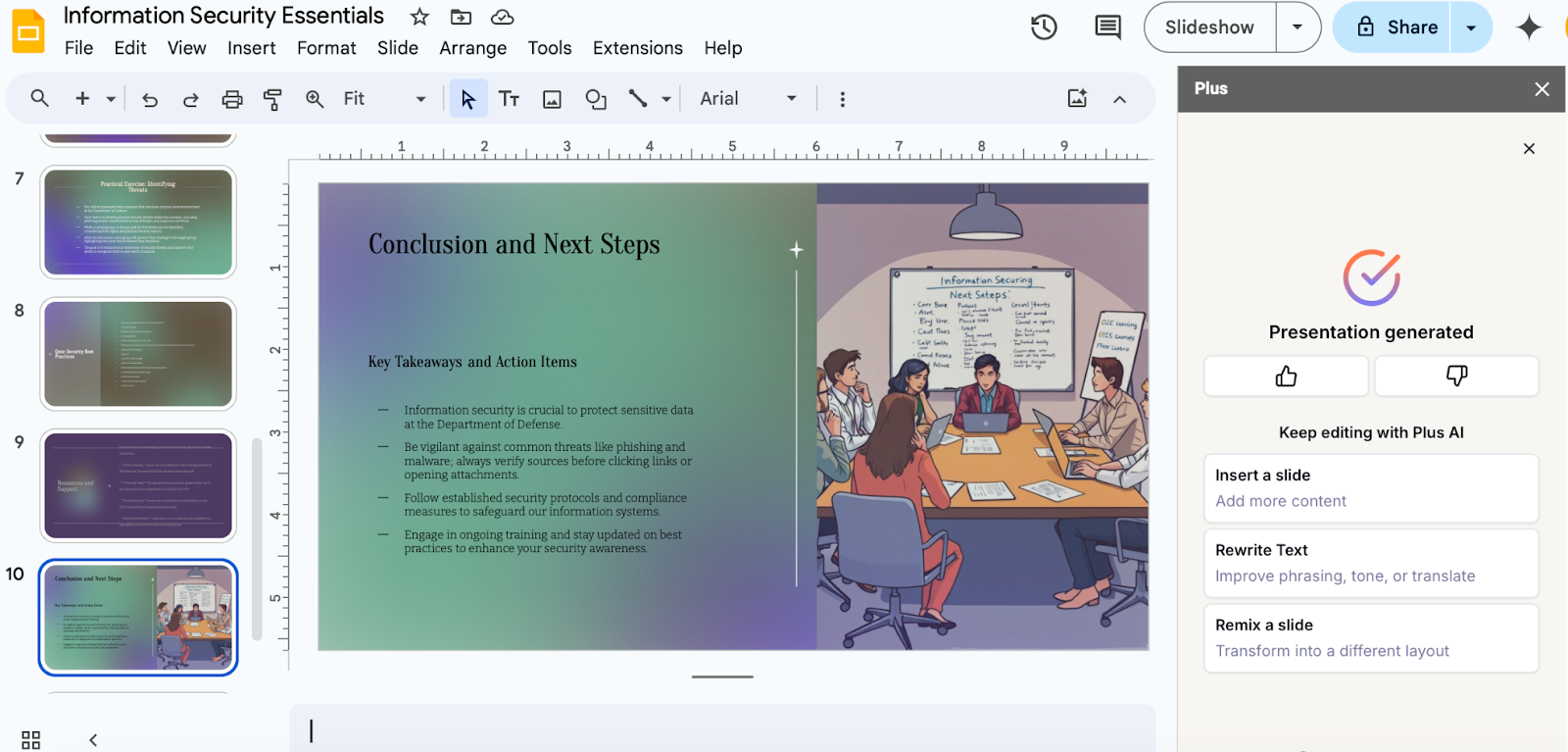
One of the more practical and uncontroversial AI image uses is in presentations. Gone are the days when you had to produce every aspect of a PowerPoint slide deck by hand. Nowadays, generative AI is able to build entire presentations — including content, formatting, and, yes, images — automatically from a user’s prompt.
For example, Plus AI is a presentation maker that works in Microsoft PowerPoint and GoogleSlides. With Plus AI, you can auto-generate entire slide decks quickly in several different ways. If you’re a novice presentation creator, or simply don’t know how to approach the first blank slide, Plus AI can convert your prompt describing the presentation topic into a complete deck. This leaves you with the much simpler task of editing the slides, or changing the AI-produced images (which are generally well-aligned with the subject matter).
Another way to use Plus AI is to upload an entire document on which you’d like to base the presentation, and the AI will create professional slides for you, images and all.
Curious to learn more about making presentations with AI? Check out Plus AI’s resource library to get started.
Design and prototyping
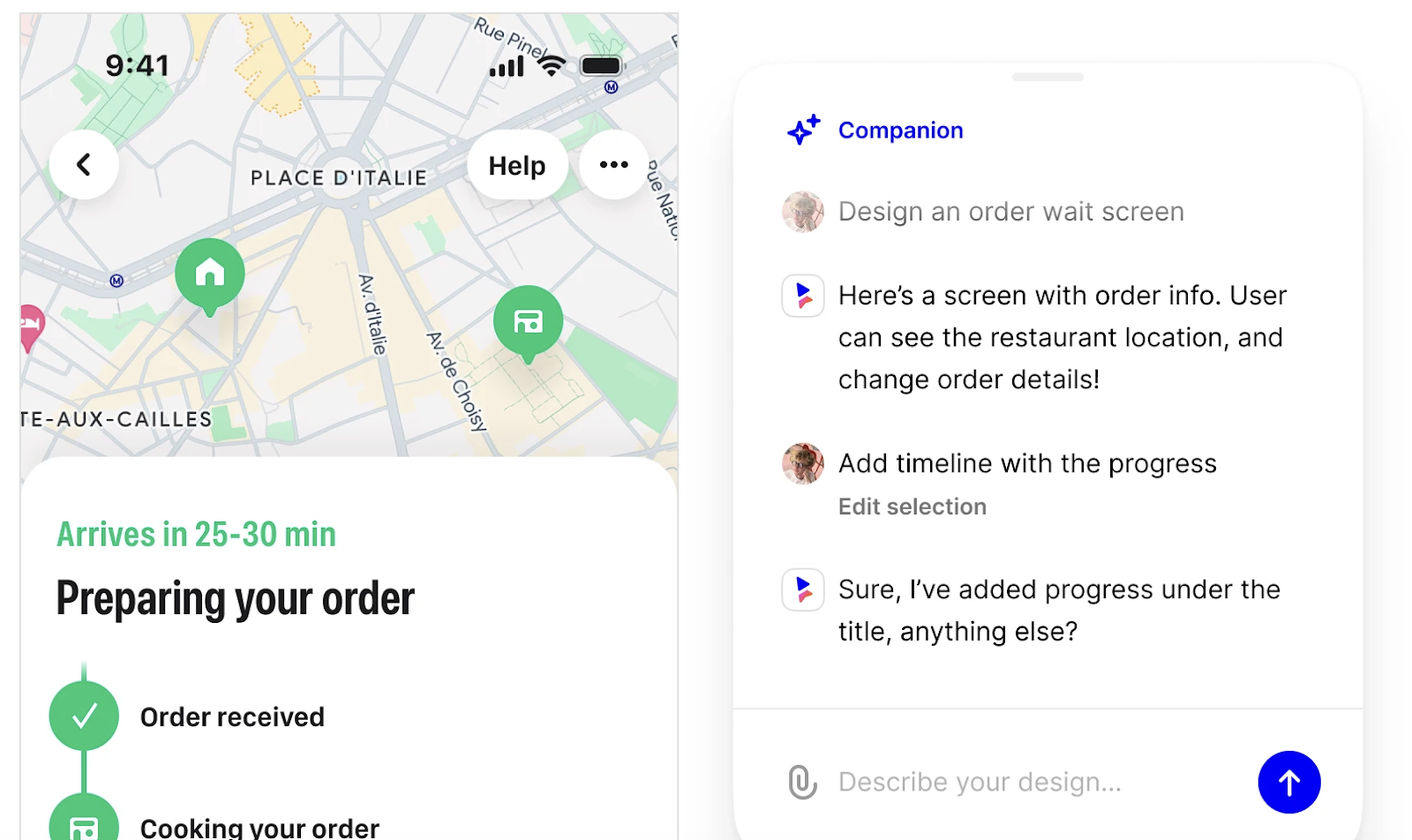
banani AI producing a UI design prototype (Source)
AI image generators integrate with product design programs so users can visualize their ideas quickly and with minimum effort by creating prototypes based on text prompts. For example, banani AI is an AI-powered prototype generator with image creation capabilities. When you prompt banani with a description of your app idea, the resulting output contains several screens with clickable buttons showing you how the interface could look, feel, and function. You can then customize the user interface with banani’s editor as you continue designing the app.
Constraints of AI image generators
For all the buzz and excitement AI image apps have generated lately, their outputs are still far from perfect and often leave a lot to be desired. Most AI programs require multiple iterations to produce a graphic that sufficiently aligns with the user’s input. Worse, these apps often struggle to draw basic elements, such as human features and text.
AI image generators often struggle to produce the desired output
If you’ve ever used Dall-e, Midjourney, or any other AI image maker, the sensation of your palm and face connecting in moments of exasperation is likely familiar. Getting these programs to produce exactly what you tell them to is usually an uphill battle, regardless of how simple and comprehensive you think your prompt is. Leaving out a tiny nuance from the input can cause AI to misinterpret the entire thing and seriously derail AI’s efforts. Meanwhile, asking to change an output image usually leaves you with a brand-new (and still imperfect) drawing.
If you’ve never had the chance to play around with prompting an AI image maker, we’ll show you an example. Here, we asked Google Gemini (which uses the proprietary Imagen 3 text-to-image model) to draw an old grandfather clock in the dusty old room of an abandoned victorian mansion:

Then, we asked Gemini to make a small tweak and have the clock to show 12:00 on the dial:
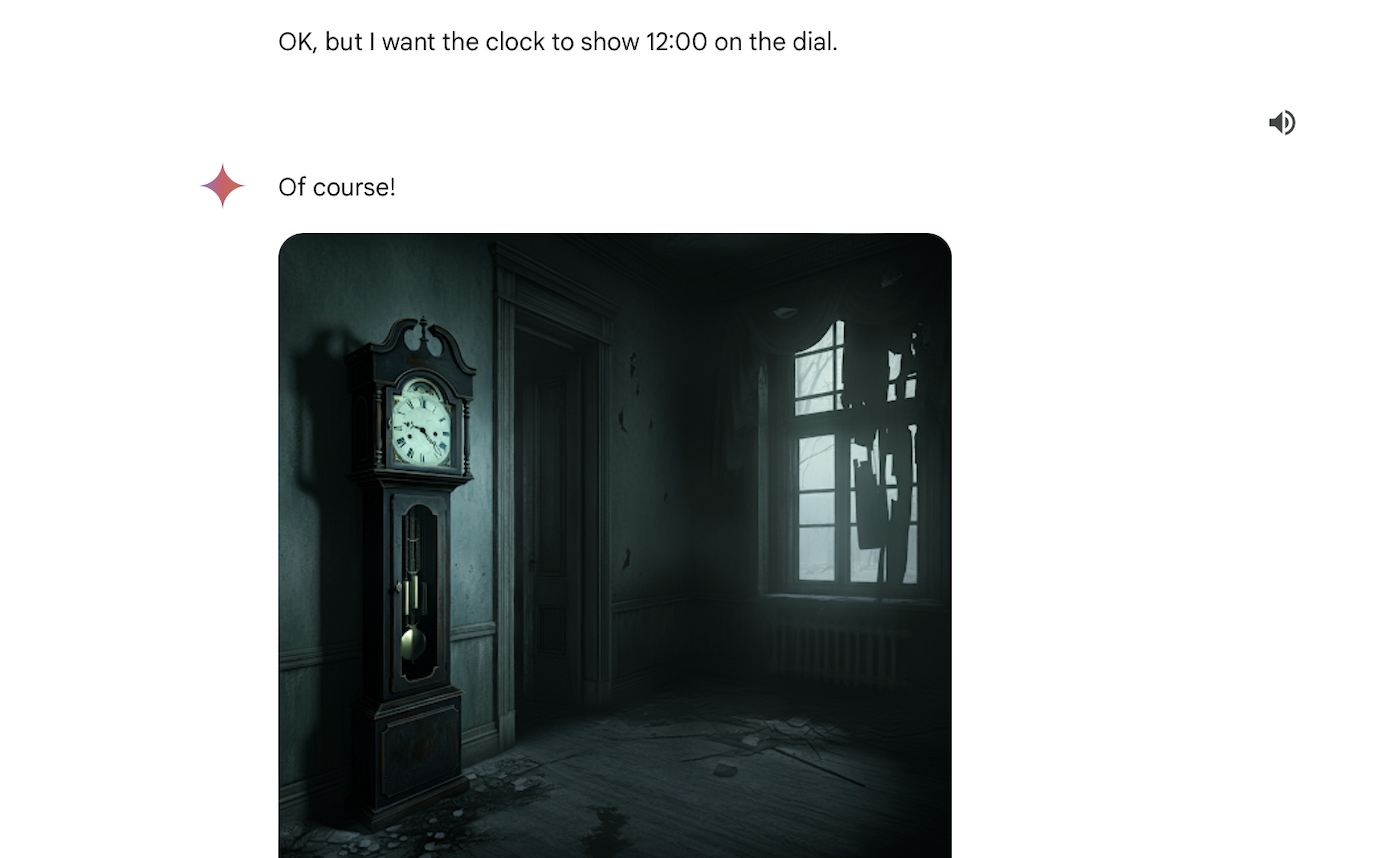
AI images often contain painfully obvious blunders
Asking AI image generators to draw people — and close-up images focusing on human features in particular — leads to some of the most egregious blunders. The reason for this shortcoming is the lack of training data needed to teach the models how to render complex features in different angles and settings.
To illustrate this drawback, we asked Stable Diffusion to “draw a man and a woman working out in the gym,” with a focus on the pair’s muscles:
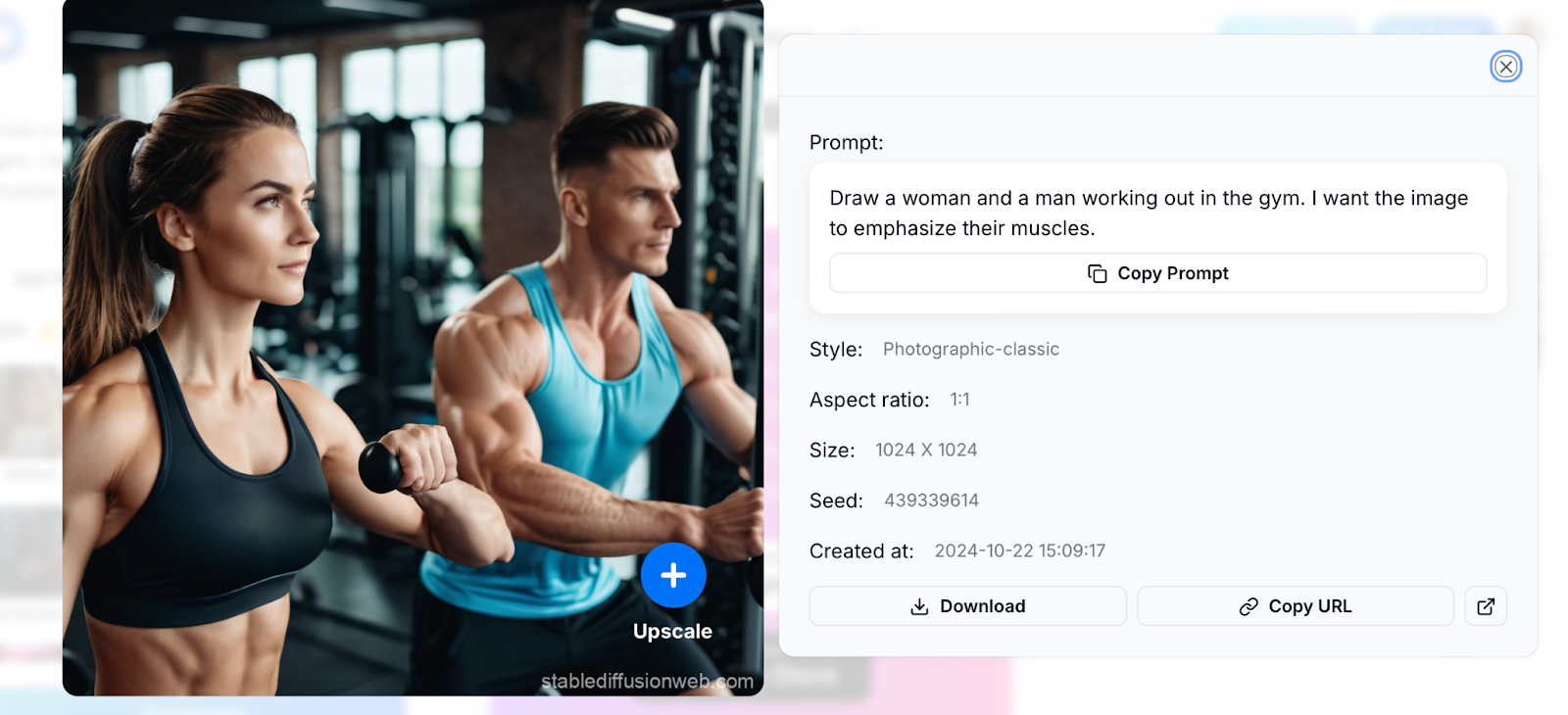
Gemini’s output to the same prompt was a bit less awkward. That said, the image still wouldn’t pass the sniff test upon closer analysis of the bodybuilders’ anatomy:

Then we took a really cheap shot and asked Stable Diffusion to draw a man and a woman shaking hands. Sure enough, the notorious hand salad emerged in the output:
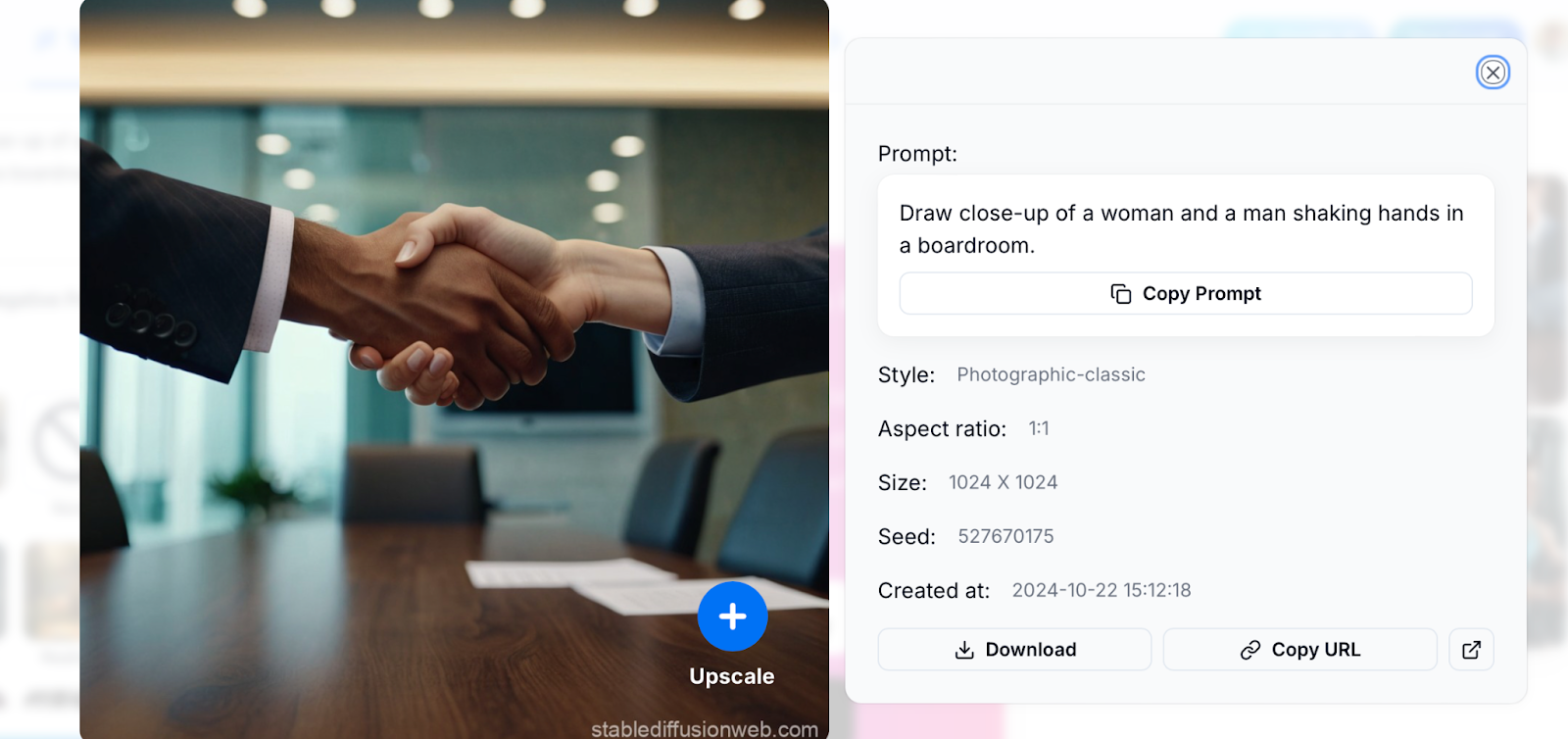
To be fair, the image above is lightyears ahead of AI’s hand renditions of years’ past:
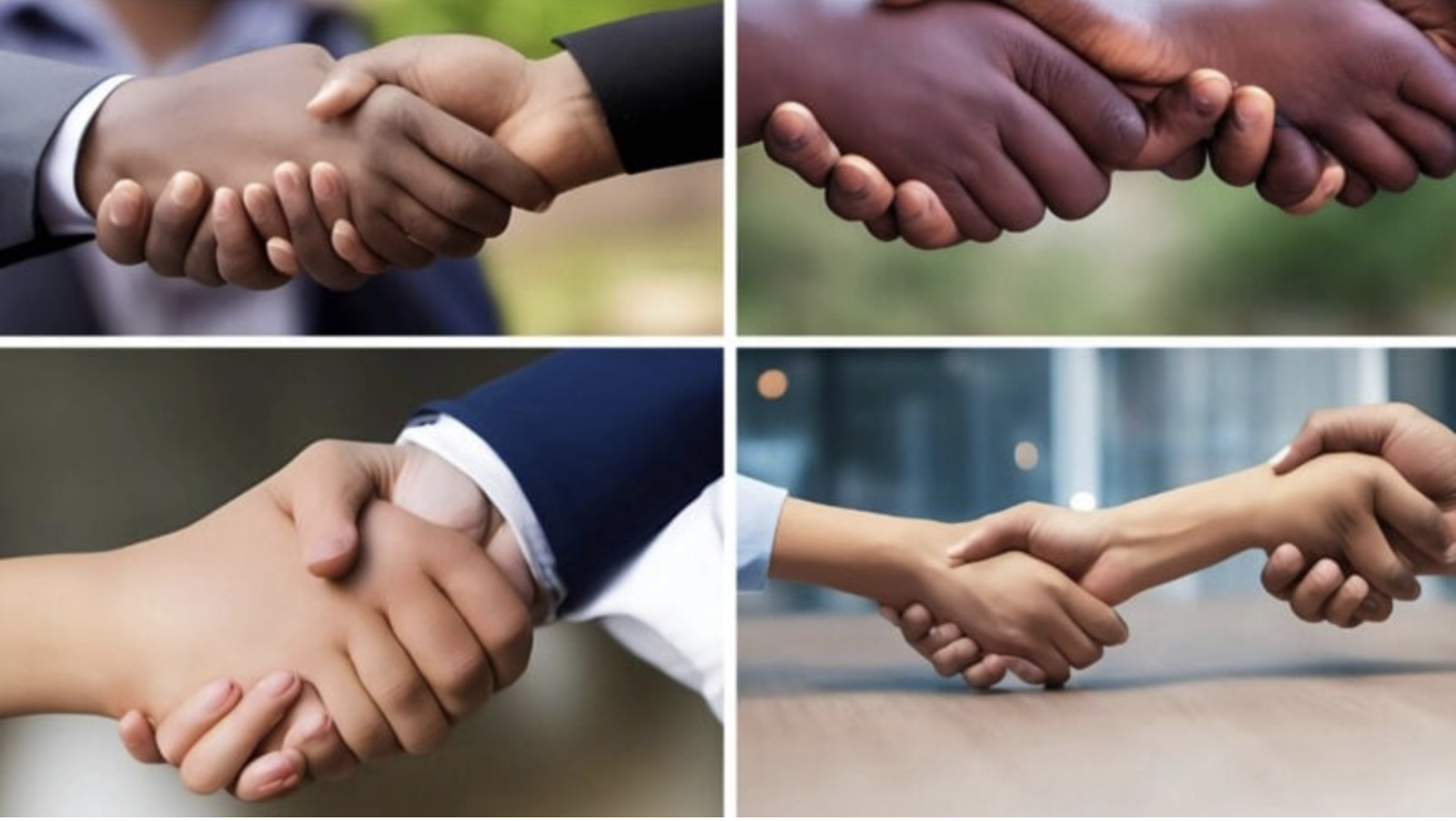
So, it’s entirely possible that the rapidly evolving technology will soon turn the corner on these blunders.
Controversies surrounding AI-generated images and art
Misinterpreted prompts and comically inaccurate human features aren’t the only issues with AI-generated graphics. As AI technology proliferates and auto-produced images flood the web, users and critics alike are concerned about intellectual property and copyright implications of images made by AI. At the same time, bad actors have used the fledgeling technology to sow misinformation for a number of nefarious purposes.
Intellectual property and copyright issues are a gray area with AI images
There are several sides to the intellectual property issue raised by AI-generated images. On one hand, creators who use AI to create graphics aren’t able to copyright these works in the US. That’s because US law only allows human-authored works to be copyrighted. On top of limitation, companies that own AI generation models usually reserve an ownership stake in the outputs their image makers produce. This means that as a creator who relies on AI to generate graphics, you won’t necessarily own the images you make.
On the other hand, the graphics drawn by AI programs may be considered derivative if they’re based on copyrighted images. You may inadvertently violate copyright laws if you get an AI model to produce a picture resembling a protected graphic asset that’s not in the public domain.
Deepfakes and misinformation have spread rapidly with AI-created images
Deepfakes — graphics that manipulate or replace a person’s appearance — have spread like wildfire with the advent of AI image models. While deepfakes have been around for years, making them with the power of artificial intelligence is infinitely faster and cheaper than doing so manually. The technology also makes deepfakes accessible to unskilled individuals who couldn’t manipulate images without AI’s help.
Deepfakes have lent themselves well to several malicious uses. They’re heavily featured in pornography, mostly with celebrities’ faces being transposed onto adult actresses’ bodies. These manipulated images have also been used to influence political outcomes and public opinion by attributing fake actions to public figures.
AI images threaten the relevance and livelihoods of human artists
AI image models devalue artistic skills and add competition to an already saturated market. With their ability to create images quickly and at a relatively low cost, AI image generators create the perception that art is easy to produce.
For the same reason, AI models have a substantial edge over their human counterparts. Instead of hiring graphic artists, marketing and content producers embrace auto-generated art as a cost-saving measure. This pivot puts immense pressure on an already saturated market, as artists face reduced job opportunities and lower wages.
Of course, many artists choose to incorporate AI into their workflows instead of shunning the new technology. But doing so poses an ethical challenge if the works are passed off as human.
FAQs about AI-generated images
Before we wrap up, we’d like to field a few common questions about AI-generated images and art.
Where does AI get its pictures from?
AI generates its pictures based on its training data, using neural network architecture and Natural Language Processing (NLP). The process differs somewhat depending on the architecture used, but generally it involves converting the user’s text input into a numerical sequence the AI can interpret contextually, then producing an image that matches the text. The AI leans on its training data — which includes countless images — to construct a likeness that resembles the user’s input.
Do I own my AI-generated images?
Whether you own your AI-generated images depends on two factors: how much effort you’ve put into creating them, and what platform you used to do so.
AI-generated images cannot be copyrighted if there is no evidence of human authorship. This means that anyone can use images you generate with AI. That said, you wade into a gray legal area if the AI-generated image is partly made by you, or comprises a larger work that you created. For example, you may be able to copyright an image that was made partially by AI and partially by you using a program like Illustrator. Likewise, you could copyright a graphic novel you wrote that contains AI-generated images (although the images themselves would not be copyrighted).
Also, consider what program you used to make the images. For example, Dall-e allows you to use images you make with its platform (which doesn’t mean you get to copyright them), but retains rights to use the outputs to train its model. On the other hand, Midjourney limits your ownership rights if you work for or own a high-earning enterprise and are not using the company’s top-tier service.
Is it ethical to use AI art generators?
Whether it’s ethical to use AI art generators depends on the intended use and the method of image creation. Obviously, it’s not ethical to use AI generators to create fake images to spread information or engage in harassment (although human-produced works are bound by the same moral standards). Likewise, you’re on shaky moral ground the art your model produces is based on copyrighted works, or even pass off your AI art as a human creation.
Crucially, you must consider the impact of AI art on human artists. Creating AI art to compete with human artists is arguably unethical — even if you come clean about using artificial intelligence in the creative process.
How do I protect my art from AI?
You can try four different techniques to protect your art from being used or replicated by an AI model:
- Watermarking and digital signatures: Adding watermarks or digital signatures to digital art can confirm your authorship and stop unauthorized use of your work.
- Cloaking digital images: Programs like Glaze use algorithms to alter the pixels in a digital image. These modifications are imperceptible to the naked eye, but prevent AI models from discerning the image.
- Opting out of AI training: Certain AI models offer opt-out options for artists. When you register your work and opt out, the AI model will not include it in its training dataset. This way, you reduce the chance of your art or style being replicated by generative AI.